SEIL: Simulation-augmented Equivariant Imitation Learning
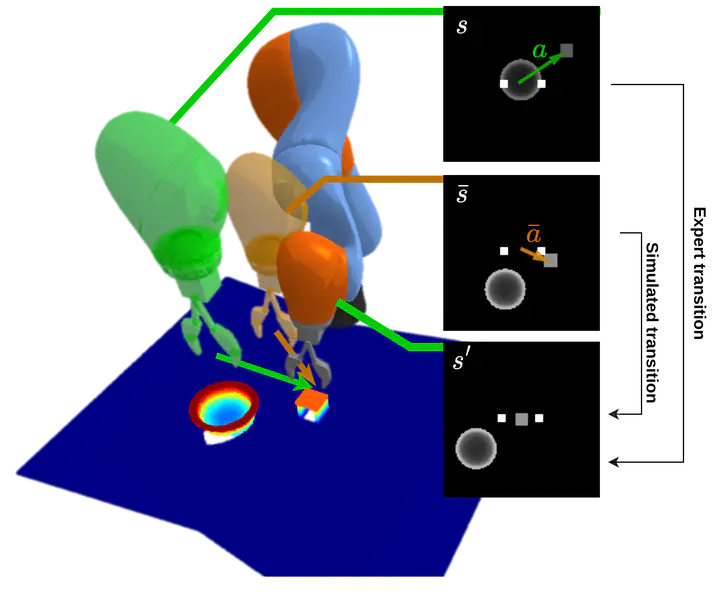
Authors
Mingxi Jia, Dian Wang, Guanang Su, David Klee, Xupeng Zhu, Robin Walters, Robert Platt
Abstract
In robotic manipulation, acquiring samples is extremely expensive because it often requires interacting with the real world. Traditional image-level data augmentation has shown the potential to improve sample efficiency in various machine learning tasks. However, image-level data augmentation is insufficient for an imitation learning agent to learn good manipulation policies in a reasonable amount of demonstrations. We propose Simulation-augmented Equivariant Imitation Learning (SEIL), a method that combines a novel data augmentation strategy of supplementing expert trajectories with simulated transitions and an equivariant model that exploits the $\mathbf{O}(2)$ symmetry in robotic manipulation. Experimental evaluations demonstrate that our method can learn non-trivial manipulation tasks within ten demonstrations and outperforms the baselines with a significant margin.
Approach
1. Equivariant Behavioral Cloning

2. Transition Simulation

Results
Simulation results
Real-world results
Experiments
Real-world tasks
- Block in Bowl
- Trash Tidying
- Shoe Packing
- Drawer Opening
Interactive close-loop behavior
- Interactive Drawers
- Interactive “Trash Tidying”
Citation
@article{jia2022seil,
title={SEIL: Simulation-augmented Equivariant Imitation Learning},
author={Jia, Mingxi; Wang, Dian; Su, Guanang; Klee, David; Zhu, Xupeng; Walters, Robin; Platt, Robert},
journal={arXiv preprint arXiv:2211.00194},
year={2022}
}